by Joche Ojeda | Jun 26, 2025 | EfCore
What is the N+1 Problem?
Imagine you’re running a blog website and want to display a list of all blogs along with how many posts each one has. The N+1 problem is a common database performance issue that happens when your application makes way too many database trips to get this simple information.
Our Test Database Setup
Our test suite creates a realistic blog scenario with:
- 3 different blogs
- Multiple posts for each blog
- Comments on posts
- Tags associated with blogs
This mirrors real-world applications where data is interconnected and needs to be loaded efficiently.
Test Case 1: The Classic N+1 Problem (Lazy Loading)
What it does: This test demonstrates how “lazy loading” can accidentally create the N+1 problem. Lazy loading sounds helpful – it automatically fetches related data when you need it. But this convenience comes with a hidden cost.
The Code:
[Test]
public void Test_N_Plus_One_Problem_With_Lazy_Loading()
{
var blogs = _context.Blogs.ToList(); // Query 1: Load blogs
foreach (var blog in blogs)
{
var postCount = blog.Posts.Count; // Each access triggers a query!
TestLogger.WriteLine($"Blog: {blog.Title} - Posts: {postCount}");
}
}
The SQL Queries Generated:
-- Query 1: Load all blogs
SELECT "b"."Id", "b"."CreatedDate", "b"."Description", "b"."Title"
FROM "Blogs" AS "b"
-- Query 2: Load posts for Blog 1 (triggered by lazy loading)
SELECT "p"."Id", "p"."BlogId", "p"."Content", "p"."PublishedDate", "p"."Title"
FROM "Posts" AS "p"
WHERE "p"."BlogId" = 1
-- Query 3: Load posts for Blog 2 (triggered by lazy loading)
SELECT "p"."Id", "p"."BlogId", "p"."Content", "p"."PublishedDate", "p"."Title"
FROM "Posts" AS "p"
WHERE "p"."BlogId" = 2
-- Query 4: Load posts for Blog 3 (triggered by lazy loading)
SELECT "p"."Id", "p"."BlogId", "p"."Content", "p"."PublishedDate", "p"."Title"
FROM "Posts" AS "p"
WHERE "p"."BlogId" = 3
The Problem: 4 total queries (1 + 3) – Each time you access blog.Posts.Count
, lazy loading triggers a separate database trip.
Test Case 2: Alternative N+1 Demonstration
What it does: This test manually recreates the N+1 pattern to show exactly what’s happening, even if lazy loading isn’t working properly.
The Code:
[Test]
public void Test_N_Plus_One_Problem_Alternative_Approach()
{
var blogs = _context.Blogs.ToList(); // Query 1
foreach (var blog in blogs)
{
// This explicitly loads posts for THIS blog only (simulates lazy loading)
var posts = _context.Posts.Where(p => p.BlogId == blog.Id).ToList();
TestLogger.WriteLine($"Loaded {posts.Count} posts for blog {blog.Id}");
}
}
The Lesson: This explicitly demonstrates the N+1 pattern with manual queries. The result is identical to lazy loading – one query per blog plus the initial blogs query.
Test Case 3: N+1 vs Include() – Side by Side Comparison
What it does: This is the money shot – a direct comparison showing the dramatic difference between the problematic approach and the solution.
The Bad Code (N+1):
// BAD: N+1 Problem
var blogsN1 = _context.Blogs.ToList(); // Query 1
foreach (var blog in blogsN1)
{
var posts = _context.Posts.Where(p => p.BlogId == blog.Id).ToList(); // Queries 2,3,4...
}
The Good Code (Include):
// GOOD: Include() Solution
var blogsInclude = _context.Blogs
.Include(b => b.Posts)
.ToList(); // Single query with JOIN
foreach (var blog in blogsInclude)
{
// No additional queries needed - data is already loaded!
var postCount = blog.Posts.Count;
}
The SQL Queries:
Bad Approach (Multiple Queries):
-- Same 4 separate queries as shown in Test Case 1
Good Approach (Single Query):
SELECT "b"."Id", "b"."CreatedDate", "b"."Description", "b"."Title",
"p"."Id", "p"."BlogId", "p"."Content", "p"."PublishedDate", "p"."Title"
FROM "Blogs" AS "b"
LEFT JOIN "Posts" AS "p" ON "b"."Id" = "p"."BlogId"
ORDER BY "b"."Id"
Results from our test:
- Bad approach: 4 total queries (1 + 3)
- Good approach: 1 total query
- Performance improvement: 75% fewer database round trips!
Test Case 4: Guaranteed N+1 Problem
What it does: This test removes any doubt by explicitly demonstrating the N+1 pattern with clear step-by-step output.
The Code:
[Test]
public void Test_Guaranteed_N_Plus_One_Problem()
{
var blogs = _context.Blogs.ToList(); // Query 1
int queryCount = 1;
foreach (var blog in blogs)
{
queryCount++;
// This explicitly demonstrates the N+1 pattern
var posts = _context.Posts.Where(p => p.BlogId == blog.Id).ToList();
TestLogger.WriteLine($"Loading posts for blog '{blog.Title}' (Query #{queryCount})");
}
}
Why it’s useful: This ensures we can always see the problem clearly by manually executing the problematic pattern, making it impossible to miss.
Test Case 5: Eager Loading with Include()
What it does: Shows the correct way to load related data upfront using Include()
.
The Code:
[Test]
public void Test_Eager_Loading_With_Include()
{
var blogsWithPosts = _context.Blogs
.Include(b => b.Posts)
.ToList();
foreach (var blog in blogsWithPosts)
{
// No additional queries - data already loaded!
TestLogger.WriteLine($"Blog: {blog.Title} - Posts: {blog.Posts.Count}");
}
}
The SQL Query:
SELECT "b"."Id", "b"."CreatedDate", "b"."Description", "b"."Title",
"p"."Id", "p"."BlogId", "p"."Content", "p"."PublishedDate", "p"."Title"
FROM "Blogs" AS "b"
LEFT JOIN "Posts" AS "p" ON "b"."Id" = "p"."BlogId"
ORDER BY "b"."Id"
The Benefit: One database trip loads everything. When you access blog.Posts.Count
, the data is already there.
Test Case 6: Multiple Includes with ThenInclude()
What it does: Demonstrates loading deeply nested data – blogs → posts → comments – all in one query.
The Code:
[Test]
public void Test_Multiple_Includes_With_ThenInclude()
{
var blogsWithPostsAndComments = _context.Blogs
.Include(b => b.Posts)
.ThenInclude(p => p.Comments)
.ToList();
foreach (var blog in blogsWithPostsAndComments)
{
foreach (var post in blog.Posts)
{
// All data loaded in one query!
TestLogger.WriteLine($"Post: {post.Title} - Comments: {post.Comments.Count}");
}
}
}
The SQL Query:
SELECT "b"."Id", "b"."CreatedDate", "b"."Description", "b"."Title",
"p"."Id", "p"."BlogId", "p"."Content", "p"."PublishedDate", "p"."Title",
"c"."Id", "c"."Author", "c"."Content", "c"."CreatedDate", "c"."PostId"
FROM "Blogs" AS "b"
LEFT JOIN "Posts" AS "p" ON "b"."Id" = "p"."BlogId"
LEFT JOIN "Comments" AS "c" ON "p"."Id" = "c"."PostId"
ORDER BY "b"."Id", "p"."Id"
The Challenge: Loading three levels of data in one optimized query instead of potentially hundreds of separate queries.
Test Case 7: Projection with Select()
What it does: Shows how to load only the specific data you actually need instead of entire objects.
The Code:
[Test]
public void Test_Projection_With_Select()
{
var blogData = _context.Blogs
.Select(b => new
{
BlogTitle = b.Title,
PostCount = b.Posts.Count(),
RecentPosts = b.Posts
.OrderByDescending(p => p.PublishedDate)
.Take(2)
.Select(p => new { p.Title, p.PublishedDate })
})
.ToList();
}
The SQL Query (from our test output):
SELECT "b"."Title", (
SELECT COUNT(*)
FROM "Posts" AS "p"
WHERE "b"."Id" = "p"."BlogId"), "b"."Id", "t0"."Title", "t0"."PublishedDate", "t0"."Id"
FROM "Blogs" AS "b"
LEFT JOIN (
SELECT "t"."Title", "t"."PublishedDate", "t"."Id", "t"."BlogId"
FROM (
SELECT "p0"."Title", "p0"."PublishedDate", "p0"."Id", "p0"."BlogId",
ROW_NUMBER() OVER(PARTITION BY "p0"."BlogId" ORDER BY "p0"."PublishedDate" DESC) AS "row"
FROM "Posts" AS "p0"
) AS "t"
WHERE "t"."row" <= 2
) AS "t0" ON "b"."Id" = "t0"."BlogId"
ORDER BY "b"."Id", "t0"."BlogId", "t0"."PublishedDate" DESC
Why it matters: This query only loads the specific fields needed, uses window functions for efficiency, and calculates counts in the database rather than loading full objects.
Test Case 8: Split Query Strategy
What it does: Demonstrates an alternative approach where one large JOIN is split into multiple optimized queries.
The Code:
[Test]
public void Test_Split_Query()
{
var blogs = _context.Blogs
.AsSplitQuery()
.Include(b => b.Posts)
.Include(b => b.Tags)
.ToList();
}
The SQL Queries (from our test output):
-- Query 1: Load blogs
SELECT "b"."Id", "b"."CreatedDate", "b"."Description", "b"."Title"
FROM "Blogs" AS "b"
ORDER BY "b"."Id"
-- Query 2: Load posts (automatically generated)
SELECT "p"."Id", "p"."BlogId", "p"."Content", "p"."PublishedDate", "p"."Title", "b"."Id"
FROM "Blogs" AS "b"
INNER JOIN "Posts" AS "p" ON "b"."Id" = "p"."BlogId"
ORDER BY "b"."Id"
-- Query 3: Load tags (automatically generated)
SELECT "t"."Id", "t"."Name", "b"."Id"
FROM "Blogs" AS "b"
INNER JOIN "BlogTag" AS "bt" ON "b"."Id" = "bt"."BlogsId"
INNER JOIN "Tags" AS "t" ON "bt"."TagsId" = "t"."Id"
ORDER BY "b"."Id"
When to use it: When JOINing lots of related data creates one massive, slow query. Split queries break this into several smaller, faster queries.
Test Case 9: Filtered Include()
What it does: Shows how to load only specific related data – in this case, only recent posts from the last 15 days.
The Code:
[Test]
public void Test_Filtered_Include()
{
var cutoffDate = DateTime.Now.AddDays(-15);
var blogsWithRecentPosts = _context.Blogs
.Include(b => b.Posts.Where(p => p.PublishedDate > cutoffDate))
.ToList();
}
The SQL Query:
SELECT "b"."Id", "b"."CreatedDate", "b"."Description", "b"."Title",
"p"."Id", "p"."BlogId", "p"."Content", "p"."PublishedDate", "p"."Title"
FROM "Blogs" AS "b"
LEFT JOIN "Posts" AS "p" ON "b"."Id" = "p"."BlogId" AND "p"."PublishedDate" > @cutoffDate
ORDER BY "b"."Id"
The Efficiency: Only loads posts that meet the criteria, reducing data transfer and memory usage.
Test Case 10: Explicit Loading
What it does: Demonstrates manually controlling when related data gets loaded.
The Code:
[Test]
public void Test_Explicit_Loading()
{
var blogs = _context.Blogs.ToList(); // Load blogs only
// Now explicitly load posts for all blogs
foreach (var blog in blogs)
{
_context.Entry(blog)
.Collection(b => b.Posts)
.Load();
}
}
The SQL Queries:
-- Query 1: Load blogs
SELECT "b"."Id", "b"."CreatedDate", "b"."Description", "b"."Title"
FROM "Blogs" AS "b"
-- Query 2: Explicitly load posts for blog 1
SELECT "p"."Id", "p"."BlogId", "p"."Content", "p"."PublishedDate", "p"."Title"
FROM "Posts" AS "p"
WHERE "p"."BlogId" = 1
-- Query 3: Explicitly load posts for blog 2
SELECT "p"."Id", "p"."BlogId", "p"."Content", "p"."PublishedDate", "p"."Title"
FROM "Posts" AS "p"
WHERE "p"."BlogId" = 2
-- ... and so on
When useful: When you sometimes need related data and sometimes don’t. You control exactly when the additional database trip happens.
Test Case 11: Batch Loading Pattern
What it does: Shows a clever technique to avoid N+1 by loading all related data in one query, then organizing it in memory.
The Code:
[Test]
public void Test_Batch_Loading_Pattern()
{
var blogs = _context.Blogs.ToList(); // Query 1
var blogIds = blogs.Select(b => b.Id).ToList();
// Single query to get all posts for all blogs
var posts = _context.Posts
.Where(p => blogIds.Contains(p.BlogId))
.ToList(); // Query 2
// Group posts by blog in memory
var postsByBlog = posts.GroupBy(p => p.BlogId).ToDictionary(g => g.Key, g => g.ToList());
}
The SQL Queries:
-- Query 1: Load all blogs
SELECT "b"."Id", "b"."CreatedDate", "b"."Description", "b"."Title"
FROM "Blogs" AS "b"
-- Query 2: Load ALL posts for ALL blogs in one query
SELECT "p"."Id", "p"."BlogId", "p"."Content", "p"."PublishedDate", "p"."Title"
FROM "Posts" AS "p"
WHERE "p"."BlogId" IN (1, 2, 3)
The Result: Just 2 queries total, regardless of how many blogs you have. Data organization happens in memory.
Test Case 12: Performance Comparison
What it does: Puts all the approaches head-to-head to show their relative performance.
The Code:
[Test]
public void Test_Performance_Comparison()
{
// N+1 Problem (Multiple Queries)
var blogs1 = _context.Blogs.ToList();
foreach (var blog in blogs1)
{
var count = blog.Posts.Count(); // Triggers separate query
}
// Eager Loading (Single Query)
var blogs2 = _context.Blogs
.Include(b => b.Posts)
.ToList();
// Projection (Minimal Data)
var blogSummaries = _context.Blogs
.Select(b => new { b.Title, PostCount = b.Posts.Count() })
.ToList();
}
The SQL Queries Generated:
N+1 Problem: 4 separate queries (as shown in previous examples)
Eager Loading: 1 JOIN query (as shown in Test Case 5)
Projection: 1 optimized query with subquery:
SELECT "b"."Title", (
SELECT COUNT(*)
FROM "Posts" AS "p"
WHERE "b"."Id" = "p"."BlogId") AS "PostCount"
FROM "Blogs" AS "b"
Real-World Performance Impact
Let’s scale this up to see why it matters:
Small Application (10 blogs):
- N+1 approach: 11 queries (≈110ms)
- Optimized approach: 1 query (≈10ms)
- Time saved: 100ms
Medium Application (100 blogs):
- N+1 approach: 101 queries (≈1,010ms)
- Optimized approach: 1 query (≈10ms)
- Time saved: 1 second
Large Application (1000 blogs):
- N+1 approach: 1001 queries (≈10,010ms)
- Optimized approach: 1 query (≈10ms)
- Time saved: 10 seconds
Key Takeaways
- The N+1 problem gets exponentially worse as your data grows
- Lazy loading is convenient but dangerous – it can hide performance problems
- Include() is your friend for loading related data efficiently
- Projection is powerful when you only need specific fields
- Different problems need different solutions – there’s no one-size-fits-all approach
- SQL query inspection is crucial – always check what queries your ORM generates
The Bottom Line
This test suite shows that small changes in how you write database queries can transform a slow, database-heavy operation into a fast, efficient one. The difference between a frustrated user waiting 10 seconds for a page to load and a happy user getting instant results often comes down to understanding and avoiding the N+1 problem.
The beauty of these tests is that they use real database queries with actual SQL output, so you can see exactly what’s happening under the hood. Understanding these patterns will make you a more effective developer and help you build applications that stay fast as they grow.
You can find the source for this article in my here
by Joche Ojeda | May 12, 2025 | C#, SivarErp
Welcome back to our ERP development series! In previous days, we’ve covered the foundational architecture, database design, and core entity structures for our accounting system. Today, we’re tackling an essential but often overlooked aspect of any enterprise software: data import and export capabilities.
Why is this important? Because no enterprise system exists in isolation. Companies need to move data between systems, migrate from legacy software, or simply handle batch data operations. In this article, we’ll build robust import/export services for the Chart of Accounts, demonstrating principles you can apply to any part of your ERP system.
The Importance of Data Exchange
Before diving into the code, let’s understand why dedicated import/export functionality matters:
- Data Migration – When companies adopt your ERP, they need to transfer existing data
- System Integration – ERPs need to exchange data with other business systems
- Batch Processing – Accountants often prepare data in spreadsheets before importing
- Backup & Transfer – Provides a simple way to backup or transfer configurations
- User Familiarity – Many users are comfortable working with CSV files
CSV (Comma-Separated Values) is our format of choice because it’s universally supported and easily edited in spreadsheet applications like Excel, which most business users are familiar with.
Our Implementation Approach
For our Chart of Accounts module, we’ll create:
- A service interface defining import/export operations
- A concrete implementation handling CSV parsing/generation
- Unit tests verifying all functionality
Our goal is to maintain clean separation of concerns, robust error handling, and clear validation rules.
Defining the Interface
First, we define a clear contract for our import/export service:
/// <summary>
/// Interface for chart of accounts import/export operations
/// </summary>
public interface IAccountImportExportService
{
/// <summary>
/// Imports accounts from a CSV file
/// </summary>
/// <param name="csvContent">Content of the CSV file as a string</param>
/// <param name="userName">User performing the operation</param>
/// <returns>Collection of imported accounts and any validation errors</returns>
Task<(IEnumerable<IAccount> ImportedAccounts, IEnumerable<string> Errors)> ImportFromCsvAsync(string csvContent, string userName);
/// <summary>
/// Exports accounts to a CSV format
/// </summary>
/// <param name="accounts">Accounts to export</param>
/// <returns>CSV content as a string</returns>
Task<string> ExportToCsvAsync(IEnumerable<IAccount> accounts);
}
Notice how we use C# tuples to return both the imported accounts and any validation errors from the import operation. This gives callers full insight into the operation’s results.
Implementing CSV Import
The import method is the more complex of the two, requiring:
- Parsing and validating the CSV structure
- Converting CSV data to domain objects
- Validating the created objects
- Reporting any errors along the way
Here’s our implementation approach:
public async Task<(IEnumerable<IAccount> ImportedAccounts, IEnumerable<string> Errors)> ImportFromCsvAsync(string csvContent, string userName)
{
List<AccountDto> importedAccounts = new List<AccountDto>();
List<string> errors = new List<string>();
if (string.IsNullOrEmpty(csvContent))
{
errors.Add("CSV content is empty");
return (importedAccounts, errors);
}
try
{
// Split the CSV into lines
string[] lines = csvContent.Split(new[] { "\r\n", "\r", "\n" }, StringSplitOptions.RemoveEmptyEntries);
if (lines.Length <= 1)
{
errors.Add("CSV file contains no data rows");
return (importedAccounts, errors);
}
// Assume first line is header
string[] headers = ParseCsvLine(lines[0]);
// Validate headers
if (!ValidateHeaders(headers, errors))
{
return (importedAccounts, errors);
}
// Process data rows
for (int i = 1; i < lines.Length; i++)
{
string[] fields = ParseCsvLine(lines[i]);
if (fields.Length != headers.Length)
{
errors.Add($"Line {i + 1}: Column count mismatch. Expected {headers.Length}, got {fields.Length}");
continue;
}
var account = CreateAccountFromCsvFields(headers, fields);
// Validate account
if (!_accountValidator.ValidateAccount(account))
{
errors.Add($"Line {i + 1}: Account validation failed for account {account.AccountName}");
continue;
}
// Set audit information
_auditService.SetCreationAudit(account, userName);
importedAccounts.Add(account);
}
return (importedAccounts, errors);
}
catch (Exception ex)
{
errors.Add($"Error importing CSV: {ex.Message}");
return (importedAccounts, errors);
}
}
Key aspects of this implementation:
- Early validation – We quickly detect and report basic issues like empty input
- Row-by-row processing – Each line is processed independently, allowing partial success
- Detailed error reporting – We collect specific errors with line numbers
- Domain validation – We apply business rules from
AccountValidator
- Audit trail – We set audit fields for each imported account
The ParseCsvLine
method handles the complexities of CSV parsing, including quoted fields that may contain commas:
private string[] ParseCsvLine(string line)
{
List<string> fields = new List<string>();
bool inQuotes = false;
int startIndex = 0;
for (int i = 0; i < line.Length; i++)
{
if (line[i] == '"')
{
inQuotes = !inQuotes;
}
else if (line[i] == ',' && !inQuotes)
{
fields.Add(line.Substring(startIndex, i - startIndex).Trim().TrimStart('"').TrimEnd('"'));
startIndex = i + 1;
}
}
// Add the last field
fields.Add(line.Substring(startIndex).Trim().TrimStart('"').TrimEnd('"'));
return fields.ToArray();
}
Implementing CSV Export
The export method is simpler, converting domain objects to CSV format:
public Task<string> ExportToCsvAsync(IEnumerable<IAccount> accounts)
{
if (accounts == null || !accounts.Any())
{
return Task.FromResult(GetCsvHeader());
}
StringBuilder csvBuilder = new StringBuilder();
// Add header
csvBuilder.AppendLine(GetCsvHeader());
// Add data rows
foreach (var account in accounts)
{
csvBuilder.AppendLine(GetCsvRow(account));
}
return Task.FromResult(csvBuilder.ToString());
}
We take special care to handle edge cases like null or empty collections, making the API robust against improper usage.
Testing the Implementation
Our test suite verifies both the happy paths and various error conditions:
- Import validation – Tests for empty content, missing headers, etc.
- Export formatting – Tests for proper CSV generation, handling of special characters
- Round-trip integrity – Tests exporting and re-importing preserves data integrity
For example, here’s a round-trip test to verify data integrity:
[Test]
public async Task RoundTrip_ExportThenImport_PreservesAccounts()
{
// Arrange
var originalAccounts = new List<IAccount>
{
new AccountDto
{
Id = Guid.NewGuid(),
AccountName = "Cash",
OfficialCode = "11000",
AccountType = AccountType.Asset,
// other properties...
},
new AccountDto
{
Id = Guid.NewGuid(),
AccountName = "Accounts Receivable",
OfficialCode = "12000",
AccountType = AccountType.Asset,
// other properties...
}
};
// Act
string csv = await _importExportService.ExportToCsvAsync(originalAccounts);
var (importedAccounts, errors) = await _importExportService.ImportFromCsvAsync(csv, "Test User");
// Assert
Assert.That(errors, Is.Empty);
Assert.That(importedAccounts.Count(), Is.EqualTo(originalAccounts.Count));
// Check first account
var firstOriginal = originalAccounts[0];
var firstImported = importedAccounts.First();
Assert.That(firstImported.AccountName, Is.EqualTo(firstOriginal.AccountName));
Assert.That(firstImported.OfficialCode, Is.EqualTo(firstOriginal.OfficialCode));
Assert.That(firstImported.AccountType, Is.EqualTo(firstOriginal.AccountType));
// Check second account similarly...
}
Integration with the Broader System
This service isn’t meant to be used in isolation. In a complete ERP system, you’d typically:
- Add a controller to expose these operations via API endpoints
- Create UI components for file upload/download
- Implement progress reporting for larger imports
- Add transaction support to make imports atomic
- Include validation rules specific to your business domain
Design Patterns and Best Practices
Our implementation exemplifies several important patterns:
- Interface Segregation – The service has a focused, cohesive purpose
- Dependency Injection – We inject the
IAuditService
rather than creating it
- Early Validation – We validate input before processing
- Detailed Error Reporting – We collect and return specific errors
- Defensive Programming – We handle edge cases and exceptions gracefully
Future Extensions
This pattern can be extended to other parts of your ERP system:
- Customer/Vendor Data – Import/export contact information
- Inventory Items – Handle product catalog updates
- Journal Entries – Process batch financial transactions
- Reports – Export financial data for external analysis
Conclusion
Data import/export capabilities are a critical component of any enterprise system. They bridge the gap between systems, facilitate migration, and support batch operations. By implementing these services with careful error handling and validation, we’ve added significant value to our ERP system.
In the next article, we’ll explore building financial reporting services to generate balance sheets, income statements, and other critical financial reports from our accounting data.
Stay tuned, and happy coding!
About Us
YouTube
https://www.youtube.com/c/JocheOjedaXAFXAMARINC
Our sites
Let’s discuss your XAF
This call/zoom will give you the opportunity to define the roadblocks in your current XAF solution. We can talk about performance, deployment or custom implementations. Together we will review you pain points and leave you with recommendations to get your app back in track
https://calendly.com/bitframeworks/bitframeworks-free-xaf-support-hour
Our free A.I courses on Udemy
by Joche Ojeda | May 5, 2025 | Uncategorized
Integration testing is a critical phase in software development where individual modules are combined and tested as a group. In our accounting system, we’ve created a robust integration test that demonstrates how the Document module and Chart of Accounts module interact to form a functional accounting system. In this post, I’ll explain the components and workflow of our integration test.
The Architecture of Our Integration Test
Our integration test simulates a small retail business’s accounting operations. Let’s break down the key components:
Test Fixture Setup
The AccountingIntegrationTests
class contains all our test methods and is decorated with the [TestFixture]
attribute to identify it as a NUnit test fixture. The Setup
method initializes our services and data structures:
[SetUp]
public async Task Setup()
{
// Initialize services
_auditService = new AuditService();
_documentService = new DocumentService(_auditService);
_transactionService = new TransactionService();
_accountValidator = new AccountValidator();
_accountBalanceCalculator = new AccountBalanceCalculator();
// Initialize storage
_accounts = new Dictionary<string, AccountDto>();
_documents = new Dictionary<string, IDocument>();
_transactions = new Dictionary<string, ITransaction>();
// Create Chart of Accounts
await SetupChartOfAccounts();
}
This method:
- Creates instances of our services
- Sets up in-memory storage for our entities
- Calls
SetupChartOfAccounts()
to create our initial chart of accounts
Chart of Accounts Setup
The SetupChartOfAccounts
method creates a basic chart of accounts for our retail business:
private async Task SetupChartOfAccounts()
{
// Clear accounts dictionary in case this method is called multiple times
_accounts.Clear();
// Assets (1xxxx)
await CreateAccount("Cash", "10100", AccountType.Asset, "Cash on hand and in banks");
await CreateAccount("Accounts Receivable", "11000", AccountType.Asset, "Amounts owed by customers");
// ... more accounts
// Verify all accounts are valid
foreach (var account in _accounts.Values)
{
bool isValid = _accountValidator.ValidateAccount(account);
Assert.That(isValid, Is.True, $"Account {account.AccountName} validation failed");
}
// Verify expected number of accounts
Assert.That(_accounts.Count, Is.EqualTo(17), "Expected 17 accounts in chart of accounts");
}
This method:
- Creates accounts for each category (Assets, Liabilities, Equity, Revenue, and Expenses)
- Validates each account using our
AccountValidator
- Ensures we have the expected number of accounts
Individual Transaction Tests
We have separate test methods for specific transaction types:
Purchase of Inventory
CanRecordPurchaseOfInventory
demonstrates recording a supplier invoice:
[Test]
public async Task CanRecordPurchaseOfInventory()
{
// Arrange - Create document
var document = new DocumentDto { /* properties */ };
// Act - Create document, transaction, and entries
var createdDocument = await _documentService.CreateDocumentAsync(document, TEST_USER);
// ... create transaction and entries
// Validate transaction
var isValid = await _transactionService.ValidateTransactionAsync(
createdTransaction.Id, ledgerEntries);
// Assert
Assert.That(isValid, Is.True, "Transaction should be balanced");
}
This test:
- Creates a document for our inventory purchase
- Creates a transaction linked to that document
- Creates ledger entries (debiting Inventory, crediting Accounts Payable)
- Validates that the transaction is balanced (debits = credits)
Sale to Customer
CanRecordSaleToCustomer
demonstrates recording a customer sale:
[Test]
public async Task CanRecordSaleToCustomer()
{
// Similar pattern to inventory purchase, but with sale-specific entries
// ...
// Create ledger entries - a more complex transaction with multiple entries
var ledgerEntries = new List<ILedgerEntry>
{
// Cash received
// Sales revenue
// Cost of goods sold
// Reduce inventory
};
// Validate transaction
// ...
}
This test is more complex, recording both the revenue side (debit Cash, credit Sales Revenue) and the cost side (debit Cost of Goods Sold, credit Inventory) of a sale.
Full Accounting Cycle Test
The CanExecuteFullAccountingCycle
method ties everything together:
[Test]
public async Task CanExecuteFullAccountingCycle()
{
// Run these in a defined order, with clean account setup first
_accounts.Clear();
_documents.Clear();
_transactions.Clear();
await SetupChartOfAccounts();
// 1. Record inventory purchase
await RecordPurchaseOfInventory();
// 2. Record sale to customer
await RecordSaleToCustomer();
// 3. Record utility expense
await RecordBusinessExpense();
// 4. Create a payment to supplier
await RecordPaymentToSupplier();
// 5. Verify account balances
await VerifyAccountBalances();
}
This test:
- Starts with a clean state
- Records a sequence of business operations
- Verifies the final account balances
Mock Account Balance Calculator
The MockAccountBalanceCalculator
is a crucial part of our test that simulates how a real database would work:
public class MockAccountBalanceCalculator : AccountBalanceCalculator
{
private readonly Dictionary<string, AccountDto> _accounts;
private readonly Dictionary<Guid, List<LedgerEntryDto>> _ledgerEntriesByTransaction = new();
private readonly Dictionary<Guid, decimal> _accountBalances = new();
public MockAccountBalanceCalculator(
Dictionary<string, AccountDto> accounts,
Dictionary<string, ITransaction> transactions)
{
_accounts = accounts;
// Create mock ledger entries for each transaction
InitializeLedgerEntries(transactions);
// Calculate account balances based on ledger entries
CalculateAllBalances();
}
// Methods to initialize and calculate
// ...
}
This class:
- Takes our accounts and transactions as inputs
- Creates a collection of ledger entries for each transaction
- Calculates account balances based on these entries
- Provides methods to query account balances and ledger entries
The InitializeLedgerEntries
method creates a collection of ledger entries for each transaction:
private void InitializeLedgerEntries(Dictionary<string, ITransaction> transactions)
{
// For inventory purchase
if (transactions.TryGetValue("InventoryPurchase", out var inventoryPurchase))
{
var entries = new List<LedgerEntryDto>
{
// Create entries for this transaction
// ...
};
_ledgerEntriesByTransaction[inventoryPurchase.Id] = entries;
}
// For other transactions
// ...
}
The CalculateAllBalances
method processes these entries to calculate account balances:
private void CalculateAllBalances()
{
// Initialize all account balances to zero
foreach (var account in _accounts.Values)
{
_accountBalances[account.Id] = 0m;
}
// Process each transaction's ledger entries
foreach (var entries in _ledgerEntriesByTransaction.Values)
{
foreach (var entry in entries)
{
if (entry.EntryType == EntryType.Debit)
{
_accountBalances[entry.AccountId] += entry.Amount;
}
else // Credit
{
_accountBalances[entry.AccountId] -= entry.Amount;
}
}
}
}
This approach closely mirrors how a real accounting system would work with a database:
- Ledger entries are stored in collections (similar to database tables)
- Account balances are calculated by processing all relevant entries
- The calculator provides methods to query this data (similar to a repository)
Balance Verification
The VerifyAccountBalances
method uses our mock calculator to verify account balances:
private async Task VerifyAccountBalances()
{
// Create mock balance calculator
var mockBalanceCalculator = new MockAccountBalanceCalculator(_accounts, _transactions);
// Verify individual account balances
decimal cashBalance = mockBalanceCalculator.CalculateAccountBalance(
_accounts["Cash"].Id,
_testDate.AddDays(15)
);
Assert.That(cashBalance, Is.EqualTo(-2750m), "Cash balance is incorrect");
// ... verify other account balances
// Also verify the accounting equation
// ...
}
The Benefits of Our Collection-Based Approach
Our redesigned MockAccountBalanceCalculator
offers several advantages:
- Data-Driven: All calculations are based on collections of data, not hardcoded values.
- Flexible: New transactions can be added easily without changing calculation logic.
- Maintainable: If transaction amounts change, we only need to update them in one place.
- Realistic: This approach closely mirrors how a real database-backed accounting system would work.
- Extensible: We can add support for more complex queries like filtering by date range.
The Goals of Our Integration Test
Our integration test serves several important purposes:
- Verify Module Integration: Ensures that the Document module and Chart of Accounts module work correctly together.
- Validate Business Workflows: Confirms that standard accounting workflows (purchasing, sales, expenses, payments) function as expected.
- Ensure Data Integrity: Verifies that all transactions maintain balance (debits = credits) and that account balances are accurate.
- Test Double-Entry Accounting: Confirms that our system properly implements double-entry accounting principles where every transaction affects at least two accounts.
- Validate Accounting Equation: Ensures that the fundamental accounting equation (Assets = Liabilities + Equity + (Revenues – Expenses)) remains balanced.
Conclusion
This integration test demonstrates the core functionality of our accounting system using a data-driven approach that closely mimics a real database. By simulating a retail business’s transactions and storing them in collections, we’ve created a realistic test environment for our double-entry accounting system.
The collection-based approach in our MockAccountBalanceCalculator
allows us to test complex accounting logic without an actual database, while still ensuring that our calculations are accurate and our accounting principles are sound.
While this test uses in-memory collections rather than a database, it provides a strong foundation for testing the business logic of our accounting system in a way that would translate easily to a real-world implementation.
Repo
egarim/SivarErp: Open Source ERP
About Us
YouTube
https://www.youtube.com/c/JocheOjedaXAFXAMARINC
Our sites
Let’s discuss your XAF
This call/zoom will give you the opportunity to define the roadblocks in your current XAF solution. We can talk about performance, deployment or custom implementations. Together we will review you pain points and leave you with recommendations to get your app back in track
https://calendly.com/bitframeworks/bitframeworks-free-xaf-support-hour
Our free A.I courses on Udemy
by Joche Ojeda | May 5, 2025 | Uncategorized
The chart of accounts module is a critical component of any financial accounting system, serving as the organizational structure that categorizes financial transactions. As a software developer working on accounting applications, understanding how to properly implement a chart of accounts module is essential for creating robust and effective financial management solutions.
What is a Chart of Accounts?
Before diving into the implementation details, let’s clarify what a chart of accounts is. In accounting, the chart of accounts is a structured list of all accounts used by an organization to record financial transactions. These accounts are categorized by type (assets, liabilities, equity, revenue, and expenses) and typically follow a numbering system to facilitate organization and reporting.
Core Components of a Chart of Accounts Module
Based on best practices in financial software development, a well-designed chart of accounts module should include:
1. Account Entity
The fundamental entity in the module is the account itself. A properly designed account entity should include:
- A unique identifier (typically a GUID in modern systems)
- Account name
- Account type (asset, liability, equity, revenue, expense)
- Official account code (often used for regulatory reporting)
- Reference to financial statement lines
- Audit information (who created/modified the account and when)
- Archiving capability (for soft deletion)
2. Account Type Enumeration
Account types are typically implemented as an enumeration:
public enum AccountType
{
Asset = 1,
Liability = 2,
Equity = 3,
Revenue = 4,
Expense = 5
}
This enumeration serves as more than just a label—it determines critical business logic, such as whether an account normally has a debit or credit balance.
3. Account Validation
A robust chart of accounts module includes validation logic for accounts:
- Ensuring account codes follow the required format (typically numeric)
- Verifying that account codes align with their account types (e.g., asset accounts starting with “1”)
- Validating consistency between account types and financial statement lines
- Checking that account names are not empty and are unique
4. Balance Calculation
One of the most important functions of the chart of accounts module is calculating account balances:
- Point-in-time balance calculations (as of a specific date)
- Period turnover calculations (debit and credit movement within a date range)
- Determining if an account has any transactions
Implementation Best Practices
When implementing a chart of accounts module, consider these best practices:
1. Use Interface-Based Design
Implement interfaces like IAccount
to define the contract for account entities:
public interface IAccount : IEntity, IAuditable, IArchivable
{
Guid? BalanceAndIncomeLineId { get; set; }
string AccountName { get; set; }
AccountType AccountType { get; set; }
string OfficialCode { get; set; }
}
2. Apply SOLID Principles
- Single Responsibility: Separate account validation, balance calculation, and persistence
- Open-Closed: Design for extension without modification (e.g., for custom account types)
- Liskov Substitution: Ensure derived implementations can substitute base interfaces
- Interface Segregation: Create focused interfaces for different concerns
- Dependency Inversion: Depend on abstractions rather than concrete implementations
3. Implement Comprehensive Validation
Account validation should be thorough to prevent data inconsistencies:
public bool ValidateAccountCode(string accountCode, AccountType accountType)
{
if (string.IsNullOrWhiteSpace(accountCode))
return false;
// Account code should be numeric
if (!accountCode.All(char.IsDigit))
return false;
// Check that account code prefix matches account type
char expectedPrefix = GetExpectedPrefix(accountType);
return accountCode.Length > 0 && accountCode[0] == expectedPrefix;
}
4. Integrate with Financial Reporting
The chart of accounts should map accounts to financial statement lines for reporting:
- Balance sheet lines
- Income statement lines
- Cash flow statement lines
- Equity statement lines
Testing the Chart of Accounts Module
Comprehensive testing is crucial for a chart of accounts module:
- Unit Tests: Test individual components like account validation and balance calculation
- Integration Tests: Verify that components work together properly
- Business Rule Tests: Ensure business rules like “assets have debit balances” are enforced
- Persistence Tests: Confirm correct database interaction
Common Challenges and Solutions
When working with a chart of accounts module, you might encounter:
1. Account Code Standardization
Challenge: Different jurisdictions may have different account coding requirements.
Solution: Implement a flexible validation system that can be configured for different accounting standards.
2. Balance Calculation Performance
Challenge: Balance calculations for accounts with many transactions can be slow.
Solution: Implement caching strategies and consider storing period-end balances for faster reporting.
3. Account Hierarchies
Challenge: Supporting account hierarchies for reporting.
Solution: Implement a nested set model or closure table for efficient hierarchy querying.
Conclusion
A well-designed chart of accounts module is the foundation of a reliable accounting system. By following these implementation guidelines and understanding the core concepts, you can create a flexible, maintainable, and powerful chart of accounts that will serve as the backbone of your financial accounting application.
Remember that the chart of accounts is not just a technical construct—it should reflect the business needs and reporting requirements of the organization using the system. Taking time to properly design this module will pay dividends throughout the life of your application.
Repo
egarim/SivarErp: Open Source ERP
About Us
YouTube
https://www.youtube.com/c/JocheOjedaXAFXAMARINC
Our sites
Let’s discuss your XAF
This call/zoom will give you the opportunity to define the roadblocks in your current XAF solution. We can talk about performance, deployment or custom implementations. Together we will review you pain points and leave you with recommendations to get your app back in track
https://calendly.com/bitframeworks/bitframeworks-free-xaf-support-hour
Our free A.I courses on Udemy
by Joche Ojeda | May 5, 2025 | Boring systems, ERP, SivarErp
Introduction
In financial accounting systems, the document module serves as the cornerstone upon which all other functionality is built. Just as physical documents form the basis of traditional accounting practices, the digital document module provides the foundation for recording, processing, and analyzing financial transactions. In this article, we’ll explore the structure and importance of the document module in a modern financial accounting system.
The Core Components
The document module consists of three essential components:
1. Documents
Documents represent the source records of financial events. These might include invoices, receipts, bank statements, journal entries, and various specialized financial documents like balance transfer statements and closing entries. Each document contains metadata such as:
- Date of the document
- Document number/reference
- Description and comments
- Document type classification
- Audit information (who created/modified it and when)
Documents serve as the legal proof of financial activities and provide an audit trail that can be followed to verify the accuracy and validity of financial records.
2. Transactions
Transactions represent the financial impact of documents in the general ledger. While a document captures the business event (e.g., an invoice), the transaction represents how that event affects the company’s financial position. A single document may generate one or more transactions depending on its complexity.
Each transaction is linked to its parent document and contains:
- Transaction date (which may differ from the document date)
- Description
- Reference to the parent document
Transactions bridge the gap between source documents and ledger entries, maintaining the relationship between business events and their financial representations.
3. Ledger Entries
Ledger entries are the individual debit and credit entries that make up a transaction. They represent the actual changes to account balances in the general ledger. Each ledger entry contains:
- Reference to the parent transaction
- Account identifier
- Entry type (debit or credit)
- Amount
- Optional references to persons and cost centers for analytical purposes
Ledger entries implement the double-entry accounting principle, ensuring that for every transaction, debits equal credits.
Why This Modular Approach Matters
The document module’s structure offers several significant advantages:
1. Separation of Concerns
By separating documents, transactions, and ledger entries, the system maintains clear boundaries between:
- Business events (documents)
- Financial impacts (transactions)
- Specific account changes (ledger entries)
This separation allows each layer to focus on its specific responsibilities without being overly coupled to other components.
2. Flexibility and Extensibility
The modular design allows for adding new document types without changing the core accounting logic. Whether handling standard invoices or specialized financial instruments, the same underlying structure applies, making the system highly extensible.
3. Robust Audit Trail
With documents serving as the origin of all financial records, the system maintains a complete audit trail. Every ledger entry can be traced back to its transaction and originating document, providing accountability and transparency.
4. Compliance and Reporting
The document-centric approach aligns with legal and regulatory requirements that mandate keeping original document records. This structure facilitates regulatory compliance and simplifies financial reporting.
Implementation Considerations
When implementing a document module, several design principles should be considered:
Interface-Based Design
Using interfaces like IDocument, ITransaction, and ILedgerEntry promotes flexibility and testability. Services operate against these interfaces rather than concrete implementations, following the Dependency Inversion Principle.
Immutability of Processed Documents
Once a document has been processed and its transactions recorded, changes should be restricted to prevent inconsistencies. Any modifications should follow proper accounting procedures, such as creating correction entries.
Versioning and Historical Records
The system should maintain historical versions of documents, especially when they’re modified, to preserve the accurate history of financial events.
Conclusion
The document module serves as the backbone of a financial accounting system, providing the structure and organization needed to maintain accurate financial records. By properly implementing this foundation, accounting systems can ensure data integrity, regulatory compliance, and flexible business operations.
Understanding the document module’s architecture helps developers and accountants alike appreciate the careful design considerations that go into building robust financial systems capable of handling the complexities of modern business operations.
Repo
egarim/SivarErp: Open Source ERP
About Us
YouTube
https://www.youtube.com/c/JocheOjedaXAFXAMARINC
Our sites
Let’s discuss your XAF
This call/zoom will give you the opportunity to define the roadblocks in your current XAF solution. We can talk about performance, deployment or custom implementations. Together we will review you pain points and leave you with recommendations to get your app back in track
https://calendly.com/bitframeworks/bitframeworks-free-xaf-support-hour
Our free A.I courses on Udemy
by Joche Ojeda | May 5, 2025 | Boring systems, ERP
After returning home from an extended journey through the United States, Greece, and Turkey, I found myself contemplating a common challenge over my morning coffee. There are numerous recurring problems in system design and ORM (Object-Relational Mapping) implementation that developers face repeatedly.
To address these challenges, I’ve decided to tackle a system that most professionals are familiar with—an ERP (Enterprise Resource Planning) system—and develop a design that achieves three critical goals:
- Performance Speed: The system must be fast and responsive
- Technology Agnosticism: The architecture should be platform-independent
- Consistent Performance: The system should maintain its performance over time
Design Decisions
To achieve these goals, I’m implementing the following key design decisions:
- Utilizing the SOLID design principles to ensure maintainability and extensibility
- Building with C# and net9 to leverage its modern language features
- Creating an agnostic architecture that can be reimplemented in various technologies like DevExpress XAF or Entity Framework
Day 1: Foundational Structure
In this first article, I’ll propose an initial folder structure that may evolve as the system develops. I’ll also describe a set of base classes and interfaces that will form the foundation of our system.
You can find all the source code for this solution in the designated repository.
The Core Layer
Today we’re starting with the core layer—a set of interfaces that most entities will implement. The system design follows SOLID principles to ensure it can be easily reimplemented using different technologies.
Base Interfaces
Here’s the foundation of our interface hierarchy:
- IEntity: Core entity interface defining the Id property
- IAuditable: Interface for entities with audit information
- IArchivable: Interface for entities supporting soft delete
- IVersionable: Interface for entities with effective dating
- ITimeTrackable: Interface for entities requiring time tracking
Service Interfaces
To complement our entity interfaces, we’re also defining service interfaces:
- IAuditService: Interface for audit-related operations
- IArchiveService: Interface for archiving operations
Repo
egarim/SivarErp: Open Source ERP
Next Steps
In upcoming articles, I’ll expand on this foundation by implementing concrete classes, developing the domain layer, and demonstrating how this architecture can be applied to specific ERP modules.
The goal is to create a reference architecture that addresses the recurring challenges in system design while remaining adaptable to different technological implementations.
Stay tuned for the next installment where we’ll dive deeper into the implementation details of our core interfaces.
About Us
YouTube
https://www.youtube.com/c/JocheOjedaXAFXAMARINC
Our sites
Let’s discuss your XAF
This call/zoom will give you the opportunity to define the roadblocks in your current XAF solution. We can talk about performance, deployment or custom implementations. Together we will review you pain points and leave you with recommendations to get your app back in track
https://calendly.com/bitframeworks/bitframeworks-free-xaf-support-hour
Our free A.I courses on Udemy
by Joche Ojeda | Apr 28, 2025 | dotnet, Uno Platform
It’s been almost a month since I left home to attend the Microsoft MVP Summit in Seattle. I’m still on the road, currently in Athens, Greece, with numerous notes for upcoming articles. While traveling makes writing challenging, I want to maintain the order of my Uno Platform series to ensure everything makes sense for readers.
In this article, we’ll dive into the structure of an Uno Platform solution. There’s some “black magic” happening behind the scenes, so understanding how everything works will make development significantly easier.
What is Uno Platform?
Before we dive into the anatomy, let’s briefly explain what Uno Platform is. Uno Platform is an open-source framework that enables developers to build cross-platform applications from a single codebase. Using C# and XAML, you can create applications that run on Windows, iOS, Android, macOS, Linux, and WebAssembly.
Root Solution Structure
An Uno Platform solution follows a specific structure that facilitates cross-platform development. Let’s break down the key components:
Main (and only) Project
The core of an Uno Platform solution is the main shared project (in our example, “UnoAnatomy”). This project contains cross-platform code shared across all target platforms and includes:
- Assets: Contains shared resources like images and icons used across all platforms. These assets may be adapted for different screen densities and platforms as needed.
- Serialization: Here is where the JsonSerializerContext lives, Since .NET 6 serialization context allows controlling how objects are serialized through the JsonSerializerContext class. It provides ahead-of-time metadata generation for better performance and reduces reflection usage, particularly beneficial for AOT compilation scenarios like Blazor WebAssembly and native apps.
- Models: Contains business model classes representing core domain entities in your application.
- Presentation: Holds UI components including pages, controls, and views. This typically includes files like
Shell.xaml.cs
and MainPage.xaml.cs
that implement the application’s UI elements and layout.
- Platforms:
- • Android: Contains the Android-specific entry point (MainActivity.Android.cs) and any other Android-specific configurations or code.
- • iOS: Contains the iOS-specific entry point (Main.iOS.cs).
- • MacCatalyst: Contains the MacCatalyst-specific entry point (Main.maccatalyst.cs).
- • BrowserWasm: Contains the Browser WASM specific configurations or code.
- • Desktop: Contains the Desktop specific configurations or code.
- Services: Contains service classes implementing business logic, data access, etc. This folder often includes subfolders like:
- Strings: the purpose of this folder is to store the localized string resources for the application so it can be translated to multiple languages.
- Styles: this folder contains the styles or color configuration for the app.
Build Configuration Files
Several build configuration files in the root of the solution control the build process:
- Directory.Build.props: Contains global MSBuild properties applied to all projects.
- Directory.Build.targets: Contains global MSBuild targets for all projects.
- Directory.Packages.props: Centralizes package versions for dependency management.
- global.json: Specifies the Uno.SDK version and other .NET SDK configurations.
The Power of Uno.Sdk
One of the most important aspects of modern Uno Platform development is the Uno.Sdk, which significantly simplifies the development process.
What is Uno.Sdk?
Uno.Sdk is a specialized MSBuild SDK that streamlines Uno Platform development by providing:
- A cross-platform development experience that simplifies targeting multiple platforms from a single project
- Automatic management of platform-specific dependencies and configurations
- A simplified build process that handles the complexity of building for different target platforms
- Feature-based configuration that enables adding functionality through the UnoFeatures property
In your project file, you’ll see <Project Sdk="Uno.Sdk">
at the top, indicating that this project uses the Uno SDK rather than the standard .NET SDK.
Key Components of the Project File
TargetFrameworks
<TargetFrameworks>net9.0-android;net9.0-ios;net9.0-maccatalyst;net9.0-windows10.0.26100;net9.0-browserwasm;net9.0-desktop</TargetFrameworks>
This line specifies that your application targets:
- Android
- iOS
- macOS (via Mac Catalyst)
- Windows (Windows 10/11 with SDK version 10.0.26100)
- WebAssembly (for browser-based applications)
- Desktop (for cross-platform desktop applications)
All of these targets use .NET 9 as the base framework.
Single Project Configuration
<OutputType>Exe</OutputType>
<UnoSingleProject>true</UnoSingleProject>
OutputType
: Specifies this project builds an executable application
UnoSingleProject
: Enables Uno’s single-project approach, allowing you to maintain one codebase for all platforms
Application Metadata
<ApplicationTitle>UnoAnatomy</ApplicationTitle>
<ApplicationId>com.companyname.UnoAnatomy</ApplicationId>
<ApplicationDisplayVersion>1.0</ApplicationDisplayVersion>
<ApplicationVersion>1</ApplicationVersion>
<ApplicationPublisher>joche</ApplicationPublisher>
<Description>UnoAnatomy powered by Uno Platform.</Description>
These properties define your app’s identity and metadata used in app stores and installation packages.
UnoFeatures
The most powerful aspect of Uno.Sdk is the UnoFeatures property:
<UnoFeatures>
Material;
Dsp;
Hosting;
Toolkit;
Logging;
Mvvm;
Configuration;
Http;
Serialization;
Localization;
Navigation;
ThemeService;
</UnoFeatures>
This automatically adds relevant NuGet packages for each listed feature:
- Material: Material Design UI components
- Dsp: Digital Signal Processing capabilities
- Hosting: Dependency injection and host builder pattern
- Toolkit: Community Toolkit components
- Logging: Logging infrastructure
- Mvvm: Model-View-ViewModel pattern implementation
- Configuration: Application configuration framework
- Http: HTTP client capabilities
- Serialization: Data serialization/deserialization
- Localization: Multi-language support
- Navigation: Navigation services
- ThemeService: Dynamic theme support
The UnoFeatures property eliminates the need to manually add numerous NuGet packages and ensures compatibility between components.
Benefits of the Uno Platform Structure
This structured approach to cross-platform development offers several advantages:
- Code Sharing: Most code is shared across platforms, reducing duplication and maintenance overhead.
- Platform-Specific Adaptation: When needed, the structure allows for platform-specific implementations.
- Simplified Dependencies: The Uno.Sdk handles complex dependency management behind the scenes.
- Consistent Experience: Ensures a consistent development experience across all target platforms.
- Future-Proofing: The architecture makes it easier to add support for new platforms in the future.
Conclusion
Understanding the anatomy of an Uno Platform solution is crucial for effective cross-platform development. The combination of shared code, platform-specific heads, and the powerful Uno.Sdk creates a development experience that makes it much easier to build and maintain applications across multiple platforms from a single codebase.
By leveraging this structure and the features provided by the Uno Platform, you can focus on building your application’s functionality rather than dealing with the complexities of cross-platform development.
In my next article in this series, we’ll dive deeper into the practical aspects of developing with Uno Platform, exploring how to leverage these structural components to build robust cross-platform applications.
Related articles
Getting Started with Uno Platform: First Steps and Configuration Choices | Joche Ojeda
My Adventures Picking a UI Framework: Why I Chose Uno Platform | Joche Ojeda
Exploring the Uno Platform: Handling Unsafe Code in Multi-Target Applications | Joche Ojeda
About Us
YouTube
https://www.youtube.com/c/JocheOjedaXAFXAMARINC
Our sites
Let’s discuss your XAF
This call/zoom will give you the opportunity to define the roadblocks in your current XAF solution. We can talk about performance, deployment or custom implementations. Together we will review you pain points and leave you with recommendations to get your app back in track
https://calendly.com/bitframeworks/bitframeworks-free-xaf-support-hour
Our free A.I courses on Udemy
by Joche Ojeda | Apr 20, 2025 | WSL
My Docker Adventure in Athens
Hello fellow tech enthusiasts!
I’m currently in Athens, Greece, enjoying a lovely Easter Sunday, when I decided to tackle a little tech project – getting Docker running on my Microsoft Surface with an ARM64 CPU. If you’ve ever tried to do this, you might know it’s not as straightforward as it sounds!
After some research, I discovered something important: there’s a difference between Docker Enterprise and Docker Community Edition (CE). While the enterprise version doesn’t support ARM64 yet, Docker CE does have versions for both ARM64 and x64 architectures. Perfect!
The WSL2 Solution
I initially tried to install Docker directly on Windows, but quickly ran into roadblocks. That’s when I decided to try the Windows Subsystem for Linux (WSL2) route instead. Spoiler alert: it worked like a charm!
While you won’t get the nice Docker Desktop UI that Windows users might be accustomed to, the command line interface through WSL2 works perfectly fine. After all, Docker was born on Linux, so running it in a Linux environment makes sense!
Step-by-Step Guide to Installing Docker CE on WSL2
Here’s how I got Docker CE up and running on my Surface using WSL2:
Step 1: Update Your Packages
First, make sure your WSL2 system is up to date:
sudo apt update && sudo apt upgrade -y
Step 2: Install Required Packages
Install the necessary packages to use HTTPS repositories:
sudo apt install -y apt-transport-https ca-certificates curl software-properties-common gnupg lsb-release
Step 3: Add Docker’s Official GPG Key
curl -fsSL https://download.docker.com/linux/ubuntu/gpg | sudo gpg --dearmor -o /usr/share/keyrings/docker-archive-keyring.gpg
Step 4: Set Up the Stable Docker Repository
echo "deb [arch=$(dpkg --print-architecture) signed-by=/usr/share/keyrings/docker-archive-keyring.gpg] https://download.docker.com/linux/ubuntu $(lsb_release -cs) stable" | sudo tee /etc/apt/sources.list.d/docker.list > /dev/null
Step 5: Update APT with the New Repository
sudo apt update
Step 6: Install Docker CE
sudo apt install -y docker-ce docker-ce-cli containerd.io
Step 7: Start the Docker Service
sudo service docker start
Step 8: Add Your User to the Docker Group
This allows you to run Docker without sudo:
sudo usermod -aG docker $USER
Step 9: Apply the Group Changes
Either log out and back in, or run:
newgrp docker
Step 10: Verify Your Installation
docker --version
docker run hello-world
Pro Tip!
If you want Docker to start automatically when you launch WSL2, add the service start command to your .bashrc or .zshrc file:
echo "sudo service docker start" >> ~/.bashrc
Final Thoughts
What started as a potentially frustrating experience turned into a surprisingly smooth process. WSL2 continues to impress me with how well it bridges the Windows and Linux worlds. If you have a Surface or any other ARM64-based Windows device and need to run Docker, I highly recommend the WSL2 approach.
Have you tried running Docker on an ARM device? What was your experience like? Let me know in the comments below!
Happy containerizing! 🐳
by Joche Ojeda | Apr 2, 2025 | Testing
In the last days, I have been dealing with a chat prototype that uses SignalR. I’ve been trying to follow the test-driven development (TDD) approach as I like this design pattern. I always try to find a way to test my code and define use cases so that when I’m refactoring or writing code, as long as the tests pass, I know everything is fine.
When doing ORM-related problems, testing is relatively easy because you can set up a memory provider, have a clean database, perform your operations, and then revert to normal. But when testing APIs, there are several approaches.
Some approaches are more like unit tests where you get a controller and directly pass values by mocking them. However, I prefer tests that are more like integration tests – for example, I want to test a scenario where I send a message to a chat and verify that the message send event was real. I want to show the complete set of moving parts and make sure they work together.
In this article, I want to explore how to do this type of test with REST APIs by creating a test host server. This test host creates two important things: a handler and an HTTP client. If you use the HTTP client, each HTTP operation (POST, GET, etc.) will be sent to the controllers that are set up for the test host. For the test host, you do the same configuration as you would for any other host – you can use a startup class or add the services you need and configure them.
I wanted to do the same for SignalR chat applications. In this case, you don’t need the HTTP client; you need the handler. This means that each request you make using that handler will be directed to the hub hosted on the HTTP test host.
Here’s the code that shows how to create the test host:
// ARRANGE
// Build a test server
var hostBuilder = new HostBuilder()
.ConfigureWebHost(webHost =>
{
webHost.UseTestServer();
webHost.UseStartup<Startup>();
});
var host = await hostBuilder.StartAsync();
//Create a test server
var server = host.GetTestServer();
And now the code for handling SignalR connections:
// Create SignalR connection
var connection = new HubConnectionBuilder()
.WithUrl("http://localhost/chathub", options =>
{
// Set up the connection to use the test server
options.HttpMessageHandlerFactory = _ => server.CreateHandler();
})
.Build();
string receivedUser = null;
string receivedMessage = null;
// Set up a handler for received messages
connection.On<string, string>("ReceiveMessage", (user, message) =>
{
receivedUser = user;
receivedMessage = message;
});
//if we take a closer look, we can see the creation of the test handler "server.CreateHandler"
var connection = new HubConnectionBuilder() .WithUrl("http://localhost/chathub", options =>
{
// Set up the connection to use the test server
options.HttpMessageHandlerFactory = _ => server.CreateHandler();
}) .Build();
Now let’s open a SignalR connection and see if we can connect to our test server:
string receivedUser = null;
string receivedMessage = null;
// Set up a handler for received messages
connection.On<string, string>("ReceiveMessage", (user, message) =>
{
receivedUser = user;
receivedMessage = message;
});
// ACT
// Start the connection
await connection.StartAsync();
// Send a test message through the hub
await connection.InvokeAsync("SendMessage", "TestUser", "Hello SignalR");
// Wait a moment for the message to be processed
await Task.Delay(100);
// ASSERT
// Verify the message was received correctly
Assert.That("TestUser"==receivedUser);
Assert.That("Hello SignalR"== receivedMessage);
// Clean up
await connection.DisposeAsync();
You can find the complete source of this example here: https://github.com/egarim/TestingSignalR/blob/master/UnitTest1.cs
by Joche Ojeda | Apr 2, 2025 | Uncategorized
It’s been a week since the Microsoft MVP Summit, and now I finally sit at Javier’s home trying to write about my trip and experience there. So let’s start!
The Journey
First, I needed to fly via Istanbul. That meant waking up around 2:00 AM to go to the airport and catch my flight at 6:00 AM. In Istanbul, I was really lucky because I was in the new airport which is huge and it has a great business lounge to wait in, so I could get some rest between my flights from Istanbul to Seattle.
I tried to sleep a little. The main problem was that the business lounge was on one side of the airport and my gate was on the other side, about 1 kilometer away. It’s a really big airport! I had to walk all that distance, and they announced the gate really late, so I only had about 15 minutes to get there—a really short time.
After that, I took my flight to the States, from Istanbul to Seattle. The route goes through the Arctic (near the North Pole)—you go up and then a little bit to the right, and then you end up in Seattle. It was a strange route; I’d never used it before. The flight was long, around 15 hours, but it wasn’t bad. I enjoyed Turkish Airlines when they use the big airplanes.
Arrival Challenges
I landed in Seattle around 6:00 PM. Then I had to go through immigration control and collect my luggage, which took almost two hours. After that, I went to the Airbnb, which was super beautiful, but I couldn’t get in because the owners had left the gate closed from the inside, and there were no lights at all, so it was impossible to enter. I waited for two hours for Javier to contact them, and after a while, it started raining, so I decided to go to a hotel. I booked a hotel for the night and took a 30-minute taxi ride. I finally went to bed on Monday at 11:00 PM, which was really late.
Day One at the Summit
The next day, I needed to drop my bags at the Airbnb and go to the MVP Summit. It was a nice experience. Javier was flying in that day and arrived around 3:00 PM, so I went to the first part alone. I missed the keynote because I had to drop off my bags and do all that stuff, so I ended up arriving around 11:00 AM.
The first person I met was Veronica, and we talked for a bit. Then I went to one of the sessions—of course, it was a Copilot session. In the afternoon, I met up with Javier, we grabbed some swag, and went to the Hub. Then I met Pablo from Argentina, and by the end of the day, I got together with Michael Washington, who I always hang out with during the MVP Summits.
Time to go home—it was a long day. We went back to the Airbnb, but didn’t do much. We just watched a TV show that our friend Hector recommended on Netflix.
Day Two: Meeting Peers
For day two, the sessions were great, but what I recall most are my meetings with specific people. When you go to the MVP Summits, you get to meet your peers. Usually, it’s like you’re good at one thing—for example, Javier and I do AI courses, and most of what we write about is general development—but there are people who really specialize.
For instance, I met the people from the Uno team, amazing people. Jerome and his team are always on the bleeding edge of .NET. We talked about the “black magic” they’ve written for their multi-target single application for Uno. It’s always nice to meet the Uno team.
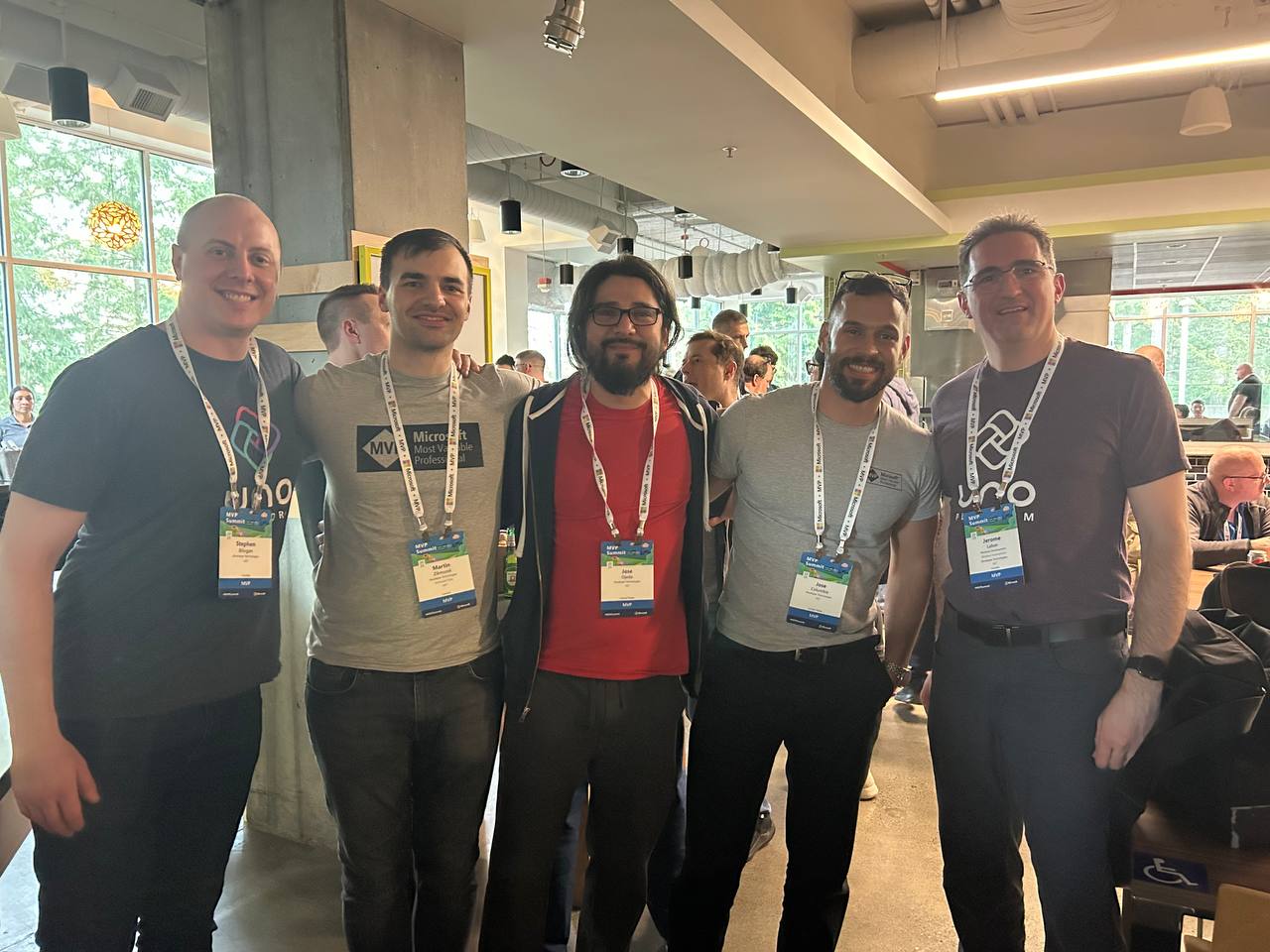
I met with Michael Washington again several times in the hallways of Microsoft, and we talked about how to redirect Microsoft AI extensions to use LLM Studio, which is kind of tricky. It’s not something you can do really easily, like with Semantic Kernel where you only need to replace the HTTP client and then you’re good to go. In LLM Studio, it’s a different trick, so I’ll write about it later.
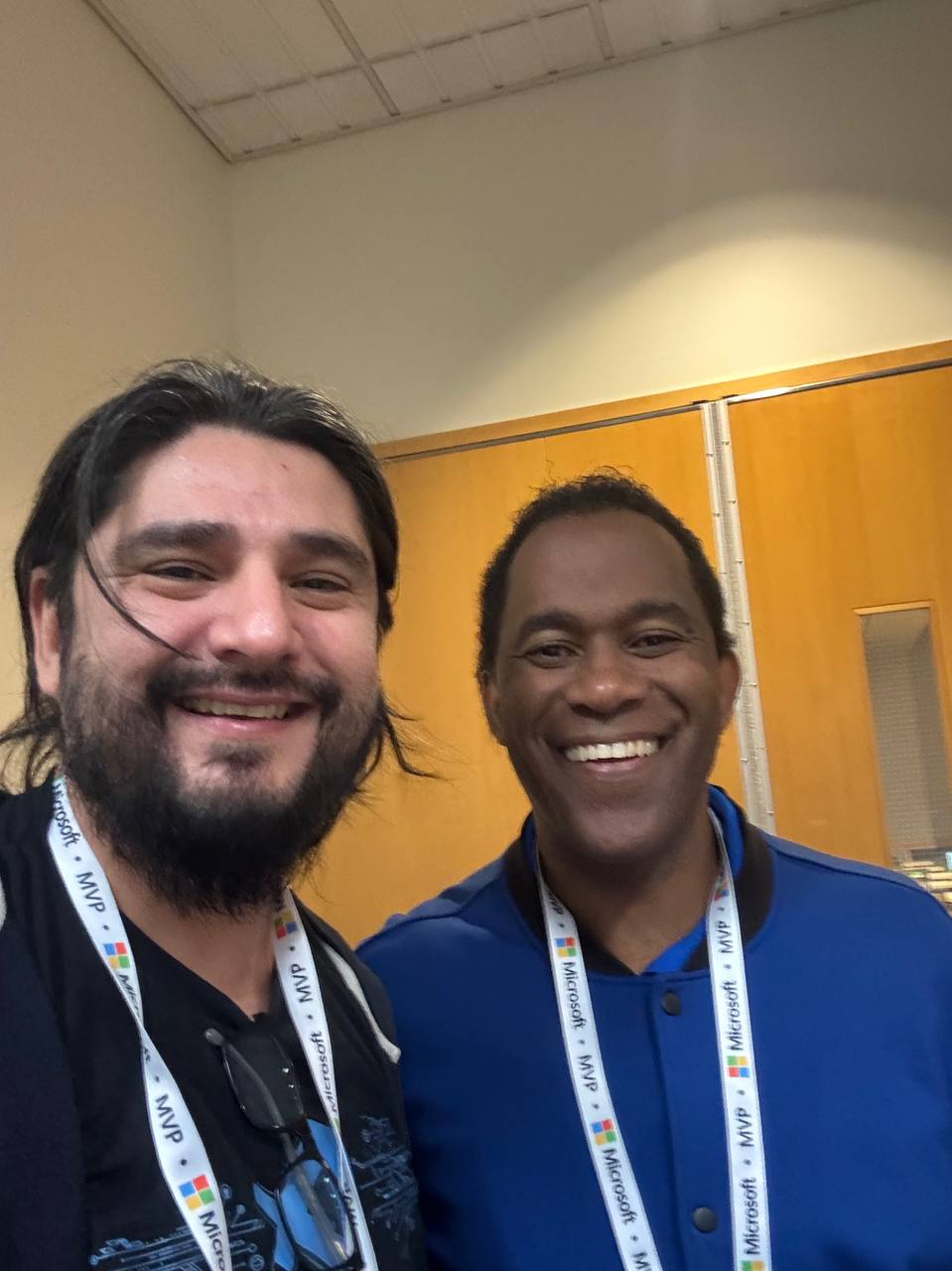
In one of the sessions, Mads Kristensen sat by my side, and I was trying to get some information from him on how to create an extension. Long ago, there was an extension from Oliver Sturm called “Instant Program Gratification” or something similar that displayed a huge congratulation message on the screen every time your compile succeeded, and if it failed, it would display something like “Hey, you need more coffee!” on the screen. I asked Mads how to achieve that with the new extension toolkit, and he explained it to me—he’s the king of extensions for Visual Studio.
Then I met someone new, Jeremy Sinclair, whom Javier introduced me to. We had one of those deep technical conversations about how Windows runs on ARM CPUs and the problems this can bring or how easy some things can be. It’s ironic because the Android architecture is usually ARM, but it doesn’t run on ARM computers because ARM computers emulate x64. We talked a lot about the challenges you might encounter and how to address them. Jeremy has managed to do it; he’s written some articles about what to expect when moving to an ARM computer. He also talked about how the future and the present for MAUI is at the moment.
He was also wearing the Ray-Ban Meta glasses, and I asked him, “Hey, how are they?” He told me they’re nice, though the battery life isn’t great, but they’re kind of fun. So I ordered a pair of Meta Ray-Ban AI glasses, and I like them so far.
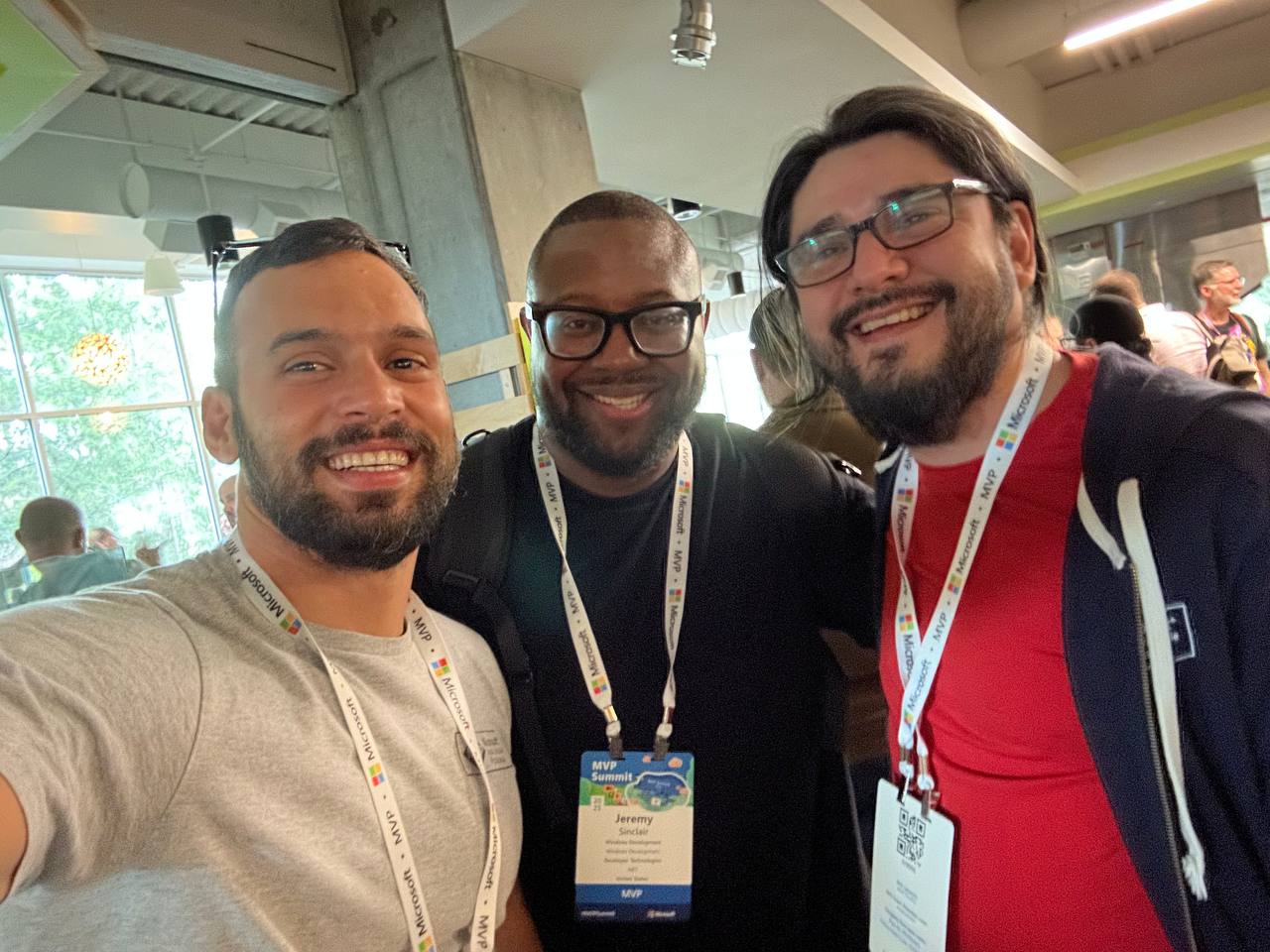
More Memorable Conversations
Another great conversation that we had with Javier was with James Montemagno. We met him in the Hub, and then we talked a lot about how we started. I’ve been a long-term fan of Merge Conflict, their podcast, and Javier introduced me to that podcast a long time ago when we met around 9 years ago. When he was traveling to work, he called me, we talked mostly about development for about one hour on his way to work, and then he told me, “Hey, I listen to this and this podcast, I listen to that and that podcast.” So I became a follower of Merge Conflict after that.
James explained all the adventures on the Xamarin team, how it went when Xamarin joined Microsoft, about the difference between Xamarin from Microsoft and Xamarin from Xamarin Forms, and how life is changing for him as more of a project manager than an advocate. So he’s kind of busy all the time, but we had this really long conversation, like 40 minutes or so. He was really open about talking about his adventure of joining Microsoft and eventually working in the MAUI team.
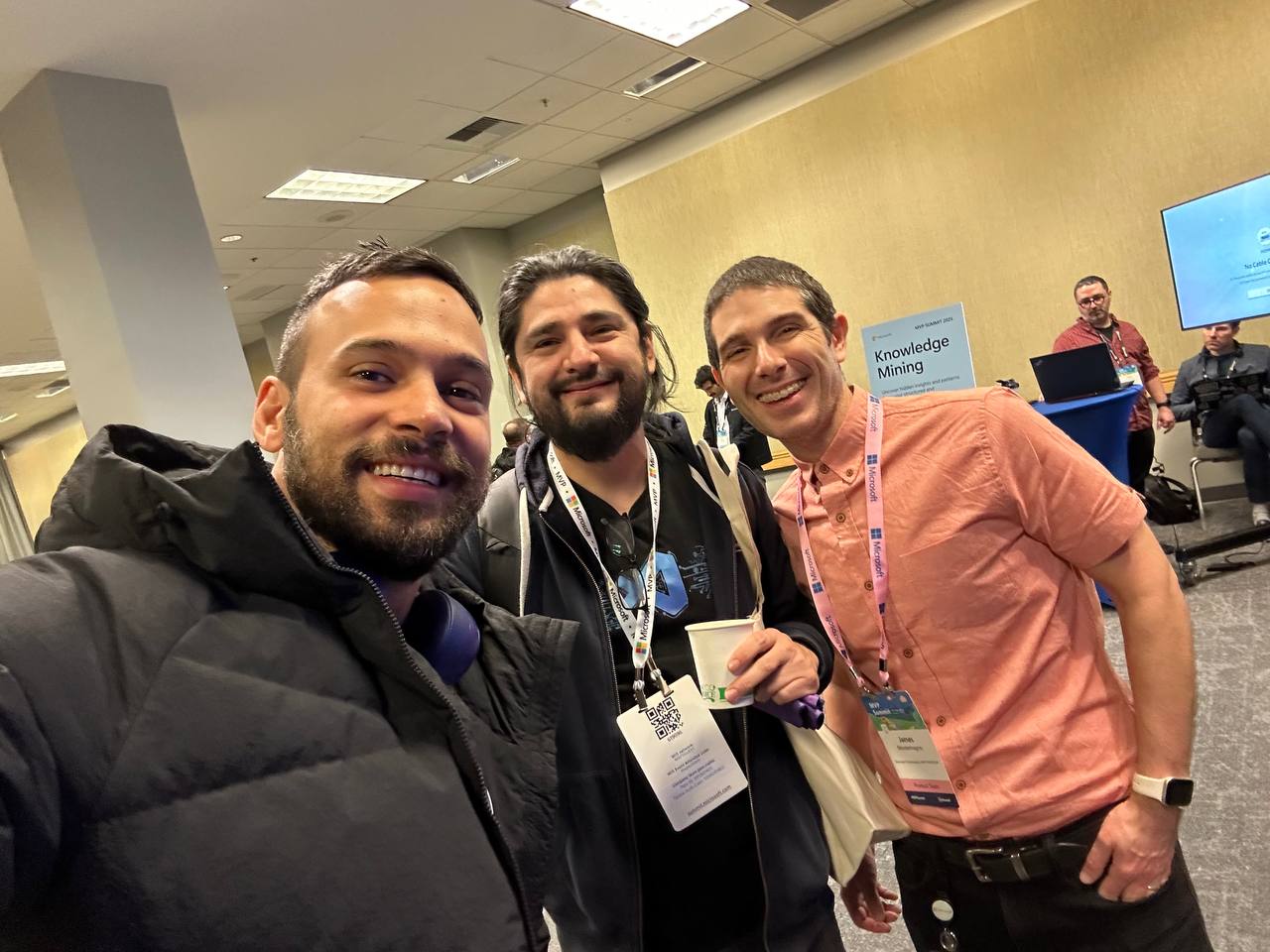
We also met David from the MAUI team, and he was so nice. Long time ago, he featured our company in the list of companies that have made apps with MAUI, and we were on the list they showed in one of the conferences. So we thanked him for that.
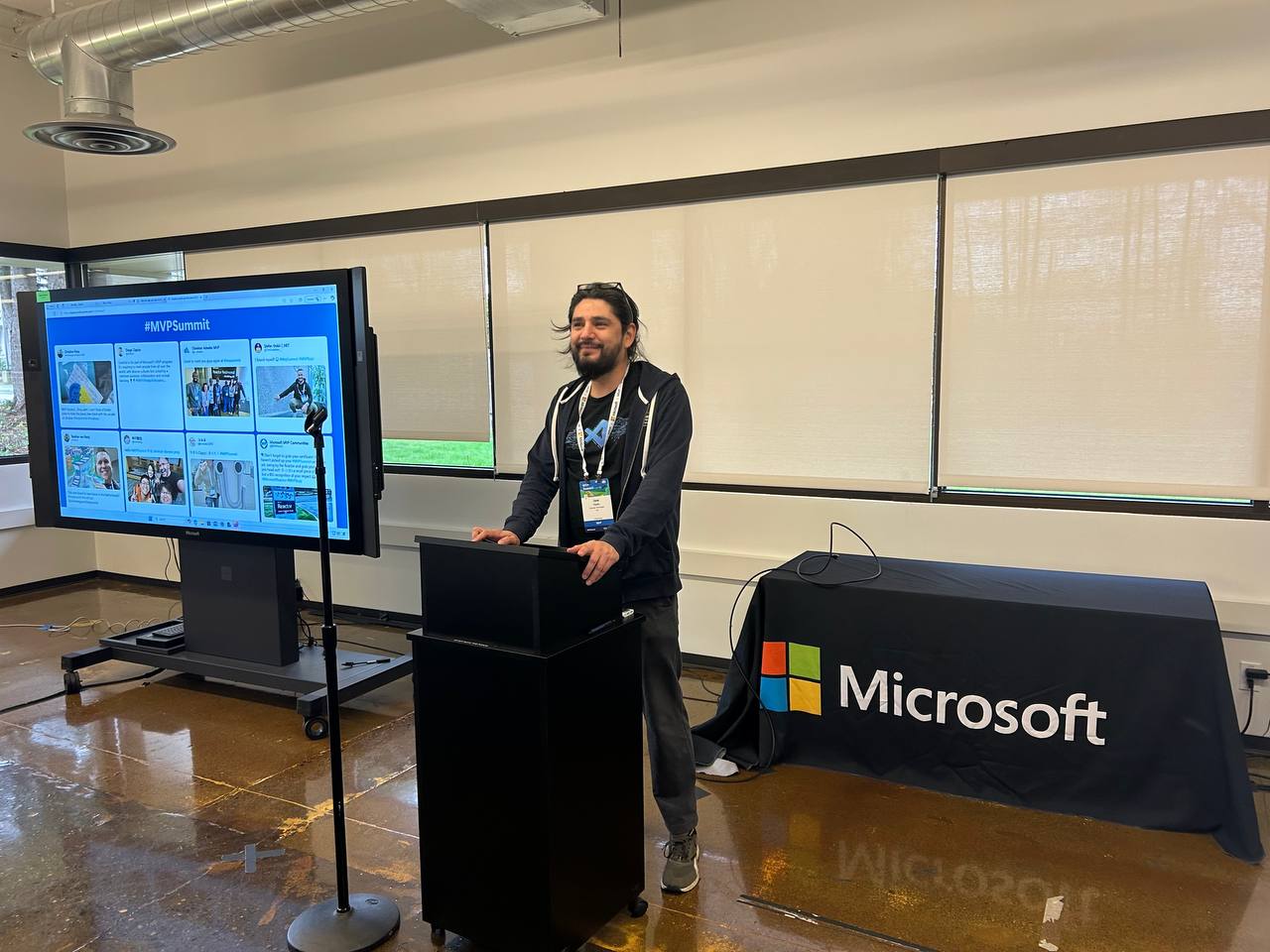
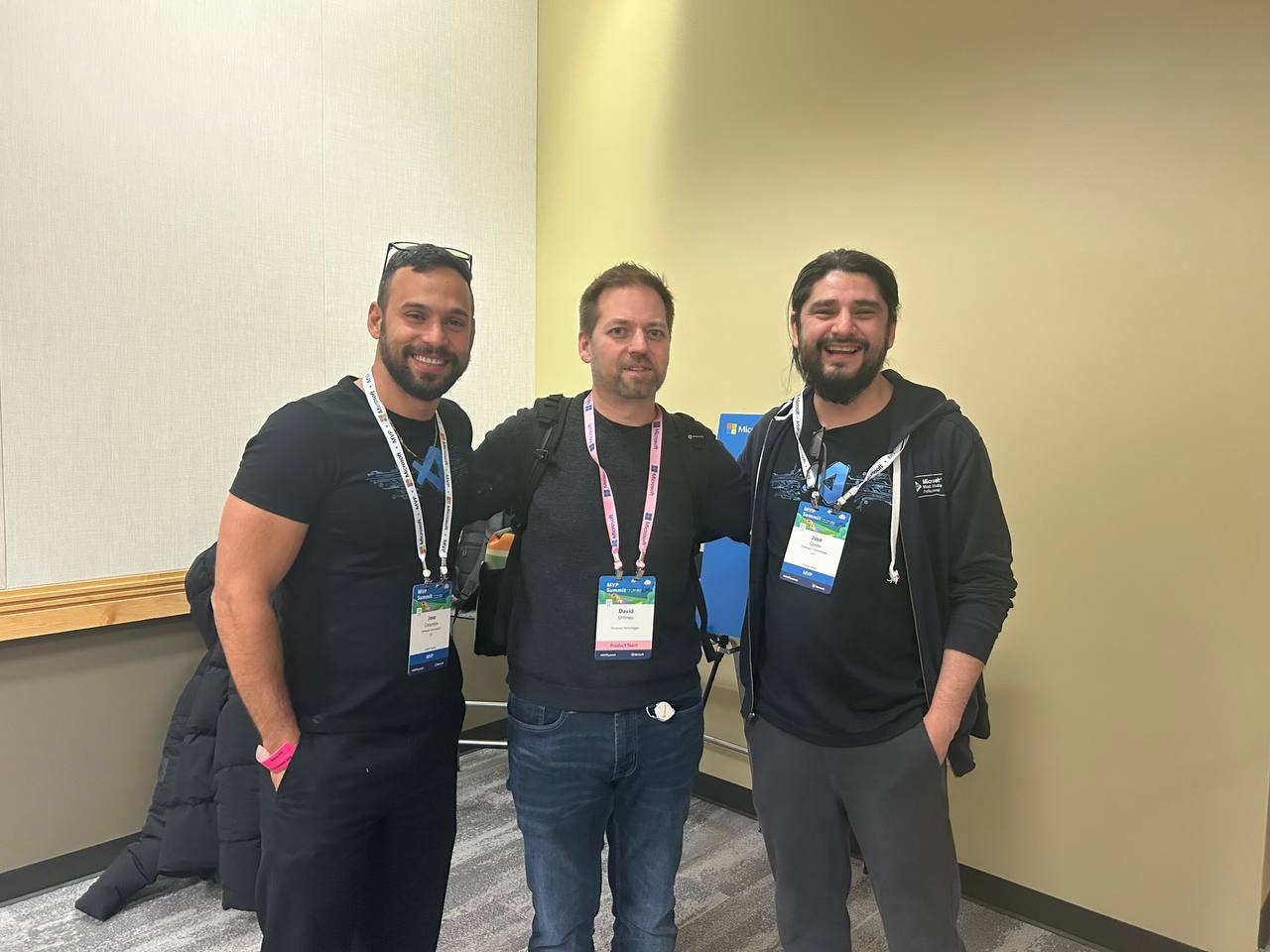
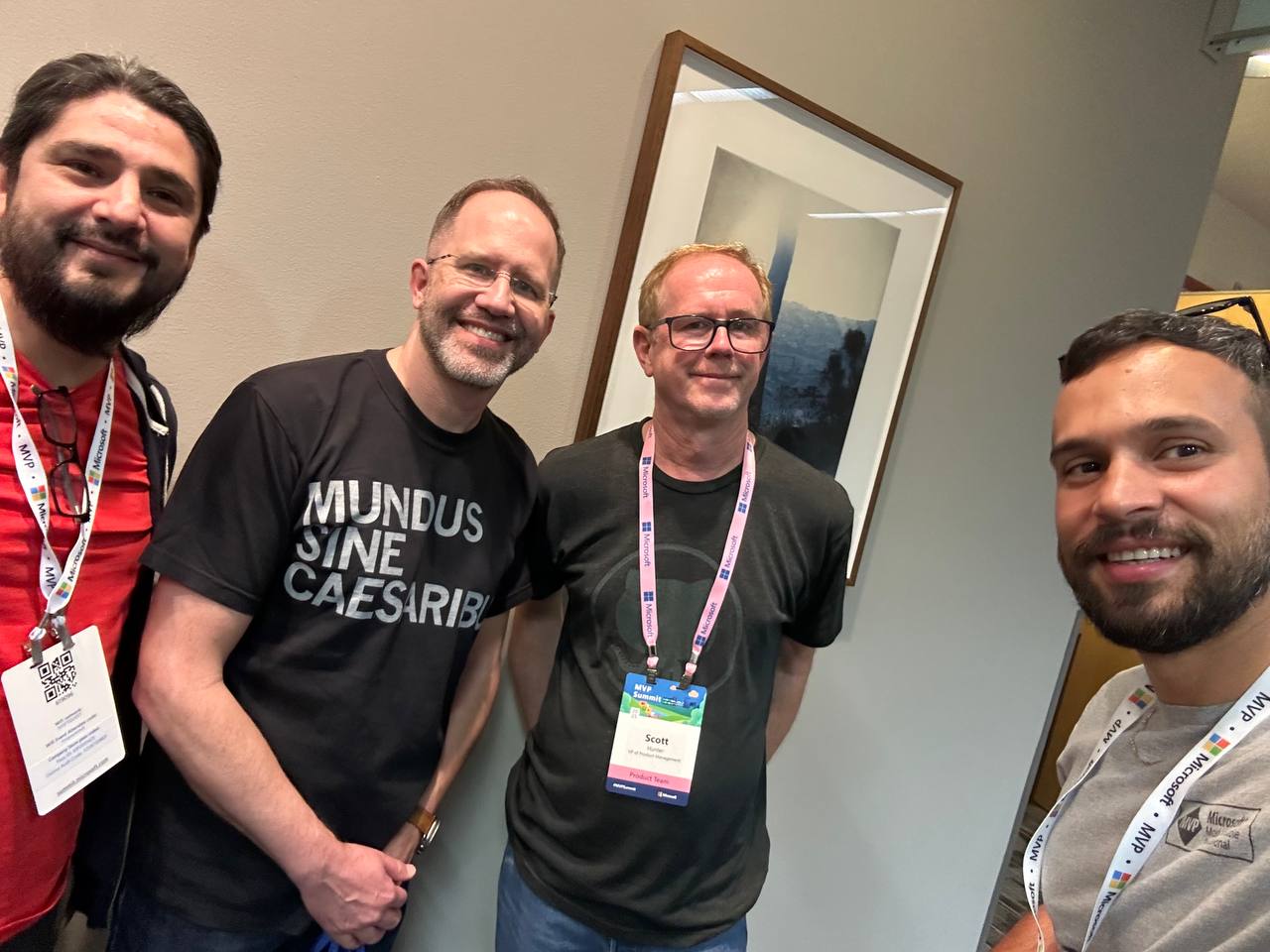
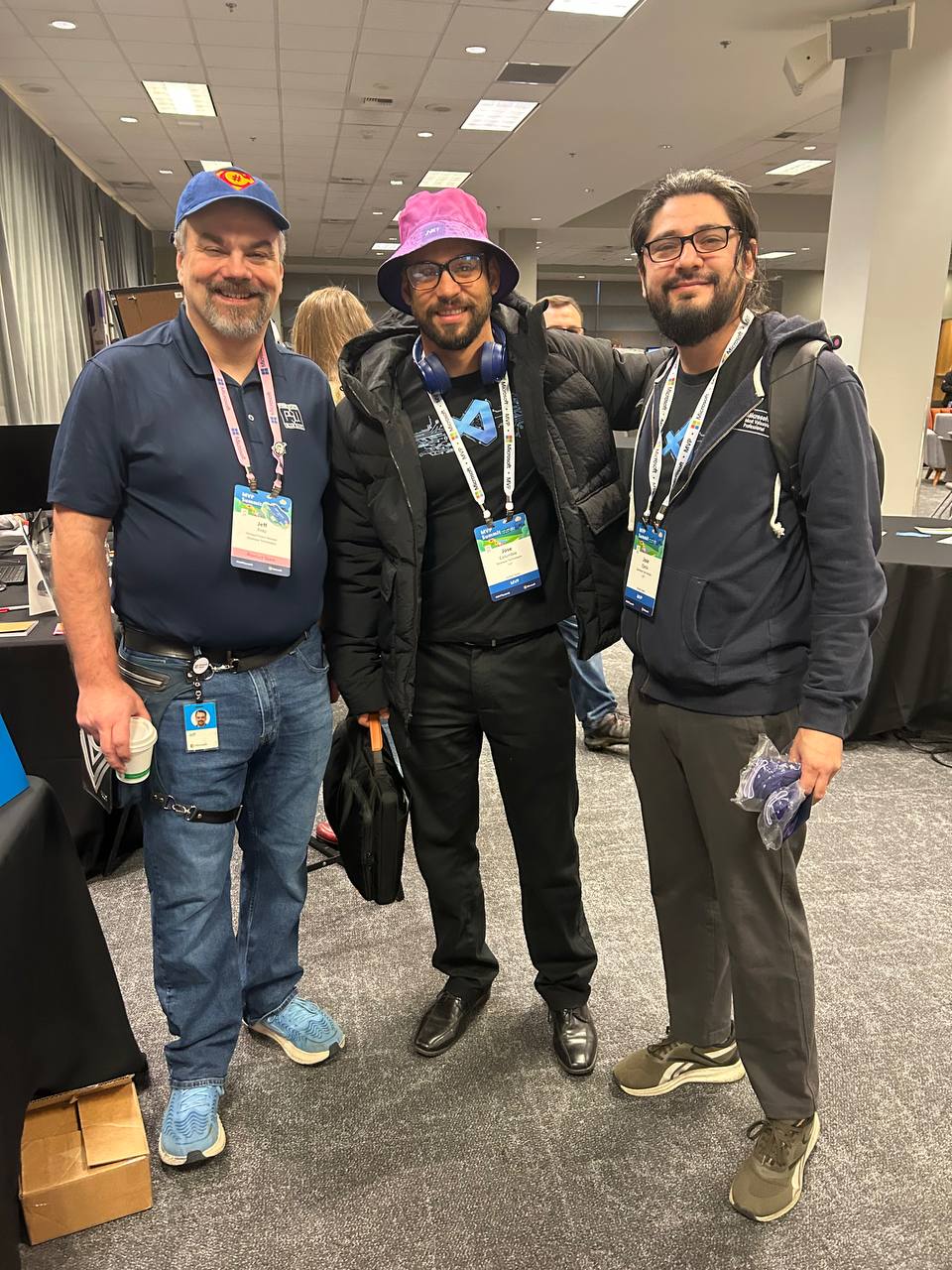
That’s everyone I met at the MVP Summit. I had a great time, and I can’t believe it’s been a year already. I’m looking forward to meeting everyone next year and seeing what we come up with during 2025!